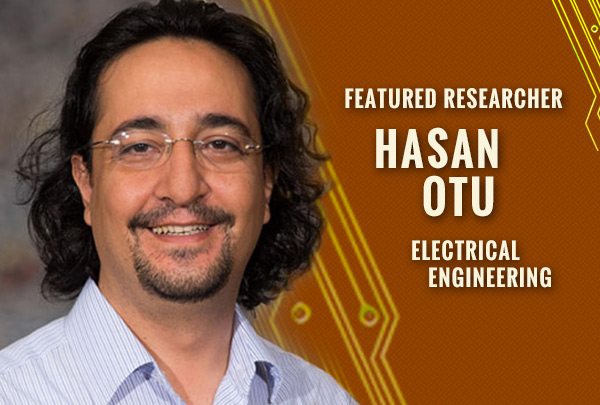
Dr. Otu holds a PhD in Electrical Engineering from the University of Nebraska and is currently a Professor in the Department of Electrical and Computer Engineering. Considered a UNL expert on Big Data, he helps us define data science, explains what big data means to his research, how he uses it, how it has helped his research and what he believes the future holds in his area.
Big Data consists of data that is either in size or complexity too large to be analyzed by standard methods and resources. I think people often fail by attributing only “size” to the concept of “big data”. “Complexity” is another important parameter. One might have data of acceptable size (i.e. possible to analyze using standard methods and resources) but this data may hold information that is too large compared to data of similar size. Such data should still be considered “big data”. For example, consider a data set that consists of 100 petabytes of a single letter “A”. This data may seem “big”, but it is not complex, hence it is not “big data”. Now, consider whole genome sequences of hundreds of individuals that add up to 100 petabytes of data. It is similar in size but far more complex than the sequence of “A”s. The latter, might be considered “big data”. Data science deals with understanding, analyzing, and comparing the structure, information, and association between different data types. Computational sciences involve mathematical and algorithmic approaches to complex problems. Data and computational sciences are the tools we use in the analysis of big data.
My research involves computational and systems biology. In my case, big data means sequence, expression, and structure measurements at the DNA, RNA and protein levels. This is further enhanced with epigenomic, metabolomic, and lipidomic data. For example, if we want to understand the mechanisms of cancer, we would like to obtain the DNA sequence of the genes, or whole genomes of cancer and normal tissue cells. This information is coupled by many individuals, cancer type, gender, population, age, etc. which immediately increases the number of biological “samples” to be sequenced by many folds. These sequencing results can be analyzed for variations such as single nucleotide polymorphisms, small InDels or translocations. However, we cannot stop there. We need to look into the activity of the genes by observing transcription at the mRNA or small RNA levels. This involves either the use of microarrays or RNA-seq to assess the abundance of tens of thousands of expressed RNA molecules. Not all expressed mRNA is translated into proteins and we may have post-translational events that impact the proteomic state of the cell. Therefore large-scale mass spectrometry, or more targeted techniques such as ELISA should provide us with information at the protein level. Only then, we might begin to see the big picture of the biological mechanisms of disease. This approach can be extended to various disease (e.g. diabetes, heart disease) or biological states (e.g. stem cells). My research involves analyzing, combining and contrasting different high throughput biological data and putting the results into context with existing knowledge, which requires a daily interaction with big data. Such an encompassing approach would require generating hundreds of gigabytes, if not terrabytes of data for a single sample. The actual “size” of this data may not seem “big” compared to, say, data we gather from social media, but I believe the complexity of it far surpasses many known data types and renders it as big data.
I use Big Data to incorporate existing external knowledge into understanding current data analysis of a specific problem. In particular, I use external data sources to understand gene interaction networks for a specific biological state. This involves gathering and analyzing large amounts of complex data from external databases. I believe today’s scientific discoveries cannot ignore the acquired knowledge and therefore I try to build frameworks that generates distilled representations of vast amounts of external knowledge. I use this Big Data analysis to understand the genomic, transcriptomic, proteomic, epigenomic, metabolomic, and lipidomic level interactions in human. Another avenue I use Big Data in, is the area of metagenomics. This involves obtaining whole genome sequences of a community of microorganisms. Microbial world is far richer and far more complex than we initially thought. Consider the fact that 90% of the cells in your body are not your cells; they are microbes. Or consider the richness and diversity of microbiology in soil and sea. Understanding the mechanism of human disease, plant biology, microbiology or many biotechnological questions requires obtaining the metagenomic sequences of microbial communities.
I use Big Data to analyze a biological or clinical state from many different angles incorporating existing knowledge. For example, in a recent study, we identified a candidate gene that simplifies and improves the efficiency of induced pluripotent stem cell generation. This required meta analysis of tens of different large data sets focused on stem cells, the oocyte and various embryonic stages. These data sets came in different formats (e.g. platform differences) and contents (e.g. transcriptomic, proteomic, etc.). In another study published less than a year ago, we presented the first detailed and high coverage whole genome sequence of a Turkish individual. This involved analysis of a few terrabytes of raw and processed data we generated for the individual and comparison of our results with whole genome and structural variation data stemming from big consortiums such as the 1000 Genomes Project.
The human whole genome consists of approximately 3.2 billion nucleotides residing on 23 chromosomes. From a computational point of view, this means analyzing 23 volumes of books that contain ~3.2 billion letters. We can infer a great deal of knowledge by just looking at the DNA of organisms, such as mutations associated with disease. Today, we can sequence the whole genome of an individual for a few thousands of dollars. In the future, I foresee everyone to obtain a copy of his or her whole genome. Considering the world’s population and the different cell types one might want to sequence in his or her body, this amounts to a significant Big Data resource for science, medicine, and industry. On the other hand, microbial metagenomic approaches alone, will produce Big Data that is a few orders of magnitude larger than what we will obtain from human whole genome data. The challenge will be to design methods and frameworks to turn this data into knowledge.
Dr. Otu obtained his B.S. degree in 1996 and his M.S. degree in 1997, both from Bogazici University, Department of Electrical and Electronics Engineering. In 2002, he graduated from the University of Nebraska-Lincoln with a Ph.D. in Electrical Engineering focusing on Bioinformatics.
He has been a faculty member at Harvard Medical School (2003 - 2012), where he was a research fellow between 2002-2003. Dr. Otu is the founding director of Bioinformatics Core at Beth Israel Deaconess Medical Center, Harvard Medical School and Associate Director of Proteomics Core at Dana Farber Harvard Cancer Center. Between 2010-2013, Dr. Otu was the founding chair of the Department of Genetics and Bioengineering at Istanbul Bilgi University.
His research interests are in the area of Bioinformatics focusing on macromolecular sequence analysis, microarrays, biomarker discovery, genetic variations and systems biology, analyzing high throughput biological data within the context of networks. Dr. Otu has published ~50 journal articles and ~30 conference proceedings, which have received over 2,500 citations. Dr. Otu has written 4 book chapters and holds 4 U.S. patents.
Experience
Academic Appointments:
•2013-present, Professor - University of Nebraska-Lincoln, Department of Electrical Engineering
•2010-2013, Assistant Prof. - Istanbul Bilgi University Department of Bioengineering, Istanbul, Turkey
•2003- 2012, Instructor of Medicine - Harvard Medical School, Boston, MA
•2002-2003, Research Fellow - Harvard Medical School, Boston, MA
•2000-2002, Instructor of EE - University of Nebraska-Lincoln, Department of Electrical Engineering
Editorial Boards and Program Committees:
•2000: Ad hoc reviewer - IEEE Transactions on Communications, Bioinformatics, Systematic Biology, Journal of Computational Chemistry, Journal of Molecular Modeling, Cancer Informatics, Journal of Molecular Evolution, BMC Bioinformatics, BMC Medical Genomics, Journal of Computational Biology, Molecular Simulation, The Computer Journal, African Journal of Biotechnology, European Journal of Human Genetics, Journal of Theoretical Biology, Acta Biotheoretica, Journal of Heredity
•2010: Associate Editor - EURASIP Journal on Bioinformatics and Systems Biology
•2011: PC Co-chair The 6th International Symposium on Health Informatics and Bioinformatics, (HIBIT)
•2012: Organizer - Workshop, “Bioinformatics Approaches for Analysis of High-throughput Biological Data”, International Centre for Genetic Engineering and Biotechnology
Selected Publications
•Otu HH*, Fortunel NO*, Ng HH*, Chen J, Mu X, Chevassut T, Li X, Joseph M, Bailey C, Hatzfeld JA, Usta F, Vega VB, Long PM, Liberman TA, Lim B. “Comment on ‘Stemness: Transcriptional Profiling of Embryonic and Adult Stem Cells’ and ‘A Stem Cell Molecular Signature’” Science 2003; 302: 393b.
•Otu HH, Sayood K. “A new sequence distance measure for phylogenetic tree construction” Bioinformatics 2003; 19:2122-2130.
•Kocabas AM, Crosby J, Ross PJ, Otu HH, Beyhan Z, Can H, Leong TW, Rosa GJM, Halgren RG, Lim B, Fernandez E and Cibelli JB. “The transcriptome of human oocytes” PNAS, 2006 103: 14027-14032.
•Steidl U, Rosenbauer F, Verhaak RGW, Gu X, Ebralidze A., Otu HH, Klippel S, Steidl C, Bruns I, Costa DB, Wagner K, Aivado M, Kobbe G, Valk PJ, Passegué E, Libermann TA, Delwel R, Tenen DG. “Essential role of Jun family transcription factors in PU.1 knockdown-induced leukemic stem cells” Nature Genetics, 2006 38(11):1269-77.
•Otu HH, Can H, Spentzos D, Nelson RG, Hanson RL, Looker HC, Knowler WC, Monroy M, Libermann TA, Karumanchi SA, Thadhani R. “Prediction of diabetic nephropathy using urine proteomic profiling 10 years prior to development of nephropathy” Diabetes Care, 2007 30:638-643.
•Otu HH, Noxerova K, Can H, Ho K, Nesbitt N, Libermann TA, Karp SJ. “Restoration of liver mass after injury requires proliferative and not embryonic transcriptional patterns” Journal of Biological Chemistry, 2007 282(15):11197-204.
•Al-Swailem AM , Shehata MM, Abu-Duhier FM, Al-Yamani EJ, Al-Busadah KA, Al-Arawi MS, Al-Khider AY, Al-Muhaimeed AN, Al-Qahtani FH, Al-Manee MM, Al-Shomrani BM, Al-Qhtani SM, Al-Harthi AS, Akdemir KC, Inan MS, Otu HH. “Sequencing, analysis, and annotation of expressed sequence tags for camelus dromedaries” PLoS ONE, 2010 5(5):e10720.
•Isci S, Jones J, Ozturk C, Otu HH. “Pathway analysis of high throughput biological data within a Bayesian Network framework” Bioinformatics, 2011 27(12):1667-1674.
•Isci S, Dogan H, Ozturk C, Otu HH. “Bayesian Network Prior: Network Analysis of Biological Data Using External Knowledge” Bioinformatics, 2014 30(6):860-867.
•Dogan H, Can H, Otu HH. “Whole Genome Sequencing of a Turkish Individual” PLoS One, 2014 9(1): e85233.
•Gonzalez-Muñoz E, Arboleda-Estudillo Y, Otu HH, Cibelli JB. “Histone chaperone ASF1A is required for maintenance of pluripotency and cellular reprogramming” Science, 2014 Jul 17. DOI:10.1126/science.1254745.
* These authors contributed equally to the work.