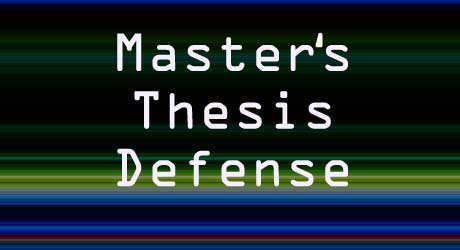
There will be four master's thesis defenses next week: Yunbo Wang on April 9 at 8:30 a.m., Amanda Swearngin on April 10 at 9 a.m., Christopher Assi on April 11 at 4 p.m., and David Anthony and Stephen Mkandawire on April 12 at 9 a.m. and 4 p.m. respectively. Included are the abstracts and locations where the defenses will be given.
Wang's Abstract:
Probabilistic QoS Analysis in Wireless Sensor Networks
118 Avery Hall
Emerging applications of wireless sensor networks (WSNs) require real-time quality of service (QoS) guarantees to be provided by the network. Traditional analysis work only focuses on the first-order statistics, such as the mean and the variance of the QoS performance. However, due to unique characteristics of WSNs, a cross-layer probabilistic analysis of QoS performance is essential. In this dissertation, a comprehensive cross-layer probabilistic analysis framework is developed to investigate the probabilistic evaluation and optimization of QoS performance provided by WSNs. In this framework, the distributions of QoS performance metrics are derived, which are natural tools to discover the probabilities to achieve given QoS requirements. Compared to first-order statistics, the distribution of these metrics reveals the relationship between the performance of QoS-based operations and the probability to achieve the performance. Using a Discrete-Time Markov queueing model in node-level analysis and fluid models in network-level analysis, the distributions of end-to-end delay, the network lifetime, and the event detection delay are then analyzed. Based on the evaluation of QoS metrics, a probabilistic optimization framework is developed to demonstrate the investigation of the optimal network and protocol parameters. Guidelines of designing networks and choosing optimal parameters for WSNs are provided using the optimization framework. Intensive testbed experiments and simulations are used to validate the accuracy of the proposed evaluation and optimization framework.
Swearngin's Abstract:
CogTool-Helper: Leveraging GUI Functional Testing Tools to Generate Predictive Human Performance Models
103C Avery Hall
Numerous tools and techniques for human performance modeling have been introduced in the field of human-computer interaction. With such tools comes the ability to model legacy applications. Models can be used to compare design ideas to existing applications, or to evaluate products against those of competitors. One such modeling tool, CogTool, allows user interface designers and analysts to mock up design ideas, demonstrate tasks, and obtain human performance predictions for those tasks. This is one step towards a simple and complete analysis process, but it still requires a large amount of manual work. Graphical user interface (GUI) testing tools are orthogonal in that they provide automated model extraction of interfaces, methods for test case generation, and test case automation; however, the resulting test cases may not mimic tasks as they are performed by experienced users.
In this thesis, we present CogTool-Helper, a tool that merges automated GUI testing with human performance modeling. It utilizes techniques from GUI testing to automatically create CogTool storyboards and models. We have designed an algorithm to find alternative methods for performing the same task, so that the UI designer or analyst can study how a user might interact with the system beyond what they have specified. We have also implemented an approach to generate functional test cases that perform tasks in a way that mimics the user. We evaluate the feasibility of our approach in a human performance regression testing scenario in LibreOffice, and show how CogTool-Helper enhances the UI designer's analysis process. Not only do the generated designs remove the need for manual design construction, but the resulting data allows new analyses that were previously not possible.
Assi's Abstract:
Data Mining of Protein Databases
347 Avery Hall
Data mining of protein databases poses special challenges because many protein databases are non-relational whereas most data mining and machine learning algorithms assume the input data to be a relational database. Protein databases are non-relational mainly because they often contain set data types. We developed new data mining algorithms that can restructure non-relational protein databases so that they become relational and amenable for various data mining and machine learning tools. We applied the new restructuring algorithms to a pancreatic protein database. After the restructuring, we also applied several classification methods, such as decision tree, neural network, and SVM classifiers and compared their accuracy in predicting whether particular pancreatic proteins are involved in pancreatic cancer.
Anthony's Abstract:
A Multi-Modal Sensing and Communication Platform for Continental Scale Migratory Bird Tracking
112 Schorr Center
This thesis presents a novel platform for tracking migratory birds on a continental scale. Cellular technology is used to augment the short-range radios that have traditionally been used in wireless sensor networks. The platform utilizes multiple sensors to enhance data collection, and create a better view of an animal's behavior. Testing methodology for this complex platforms is presented. The platform is used in a series of simulations and field tests to demonstrate its merit and capabilities.
Mkandawire's Abstract:
Improving Backup and Restore Performance for Deduplication-based Cloud Backup Services
347 Avery Hall
Data mining of protein databases poses special challenges because many protein databases are non-relational whereas most data mining and machine learning algorithms assume the input data to be a relational database. Protein databases are non-relational mainly because they often contain set data types. We developed new data mining algorithms that can restructure non-relational protein databases so that they become relational and amenable for various data mining and machine learning tools. We applied the new restructuring algorithms to a pancreatic protein database. After the restructuring, we also applied several classification methods, such as decision tree, neural network, and SVM classifiers and compared their accuracy in predicting whether particular pancreatic proteins are involved in pancreatic cancer.