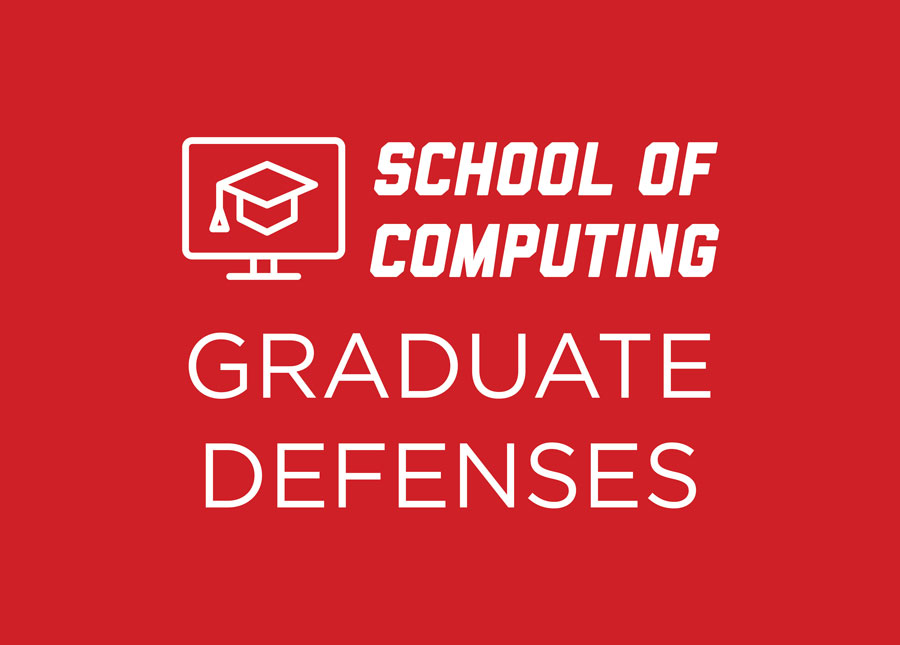
M.S. Project Defense: Thuy An
December 1, 2021
2–3 PM CST
Join Zoom Meeting: https://unl.zoom.us/j/93597957479
Meeting ID: 935 9795 7479
“Computational Solutions to Exosomal miRNA Biomarker Detection in Pancreatic Cancer”
Abstract: Pancreatic cancer is the fourth leading cause of cancer death in the United States and the 5-year survival rate is only 5% to 10%. There are only a few non-specific symptoms associated with the early stage cancer, therefore most patients are diagnosed in a late stage. Due to the lack of effective treatments on pancreatic cancer and the fact that the early stage has a 39% 5-year survival rate, the biggest hope to control this disease is early detection. Therefore, discovery of effective and reliable non-invasive biomarkers for early detection of pancreatic cancer has been a major topic in the research field. Very recently, exosomal miRNAs have become promising candidates of diagnostic markers due to the facts that 1) such small non-coding RNA are stably present in the tissue and can get into blood circulation via exosome packaging which protects them from enzymatic degradation; 2) cancer cells, even at their early stages, may secrete up to tenfold more exosomes than normal cells and some disease-associated miRNAs can get into blood stream; 3) circulating exosomal miRNAs may carry early signals of cancers. With the goal to facilitate cancer detection, in this study, we have developed an integrated computational approach that leverages advanced genomics and bioinformatics to identify exosomal miRNAs that can be promising early detection biomarkers in pancreatic cancer. First, we have analyzed large-scale small RNA microarray data collected from the GEO database to identify miRNA candidates that are differentially-expressed in cancer versus healthy control. Then we have explored several classification models to identify the most effective signature of miRNAs that can well differentiate cancer from normal based on the expression profiles. Through a series of comparisons and validations, the support vector machine-based classifier has achieved the best performance among all. A combination of five significantly-expressed miRNAs (hsa-miR-125a-3p, hsa-miR-6893-5p, hsa-miR-125b-1-3p, hsa-miR-6075, and hsa-miR-4294) achieved the best accuracy and AUC of 97.59% and 99.70%, respectively, which is highly promising. In order to explore the molecular determinants in miRNA secretion to further guide the non-invasive biomarker detection in the blood stream, in the second part of this project, we focused on sequence analysis of exosomal miRNAs for motif detection. Particularly, a graph-based motif-finding algorithm previously developed in our lab has been applied for this purpose. As a result, the motif [CUG][AU]G[UG] was found highly enriched in miRNAs associated with cancer exosomes. Knowing such properties is highly useful to guide a more targeted search in contrast to the profiling-based discovery where most of the detected miRNAs are highly abundant but likely disease irrelevant. In summary, our study has presented a new data-driven strategy that can potentially advance the biomedical research in biomarker discovery. Particularly, we have demonstrated that circulating exosomal miRNAs can be used as promising stable non-invasive biomarkers for early diagnosis of pancreatic cancer.
Committee Members:
Juan Cui, Chair
Jitender Deogun, Co-Chair
Massimiliano Pierobon
M.S. Thesis Defense: Lei Yu
December 2, 2021
3:30–4:30 PM CST
Zoom: https://unl.zoom.us/j/93959397461
Meeting ID: 939 5939 7461
“Information Extraction and Classification on Journal Papers”
Digitized documents have become an omnipresent medium of information. A plethora of scholarly documents on the web is excessively being increased. Most of the scientific literature is stored in Portable Document Format (PDF). PDF documents hold a complex structure due to which their comprehension and extraction of useful information from them is a challenging task. In this regard, research community has been proposing different rule based and machine learning based techniques in the past several years. We believe that accurate and efficient information extraction form the PDF files is an important issue as major portion of scholarly literature is stored in PDF.
To help a soil science team from the United States Department of Agriculture (USDA) build a queryable journal paper system, we used web crawler with Python to download journal papers on soil science from the digital library to provide users with papers they are interested in. To extract useful information including authors, journal, publish date, abstract, DOI, journal type, experiment location and key words in papers and highlight the paper characteristics in data system, we applied named entity recognition to extract authors and location of experiments, table analysis to extract tables in the paper. The named entity recognition technique is used to extract authors and experiment location. And the table analysis is used to store the tables from the journal paper in a computer queryable form. Text analysis is applied to figure out the parts of interest, and stored them in the database to save time. We used traditional machine learning techniques including logistic regression, support vector machine, decision tree, naive bayes, k-nearest neighbors, random forest, ensemble modeling, and neural networks in text analysis and compare the advantages of these approaches in the end.
Committee:
Prof. Stephen D. Scott
Prof. Vinodchandran Variyam
Prof. Ashok Samal