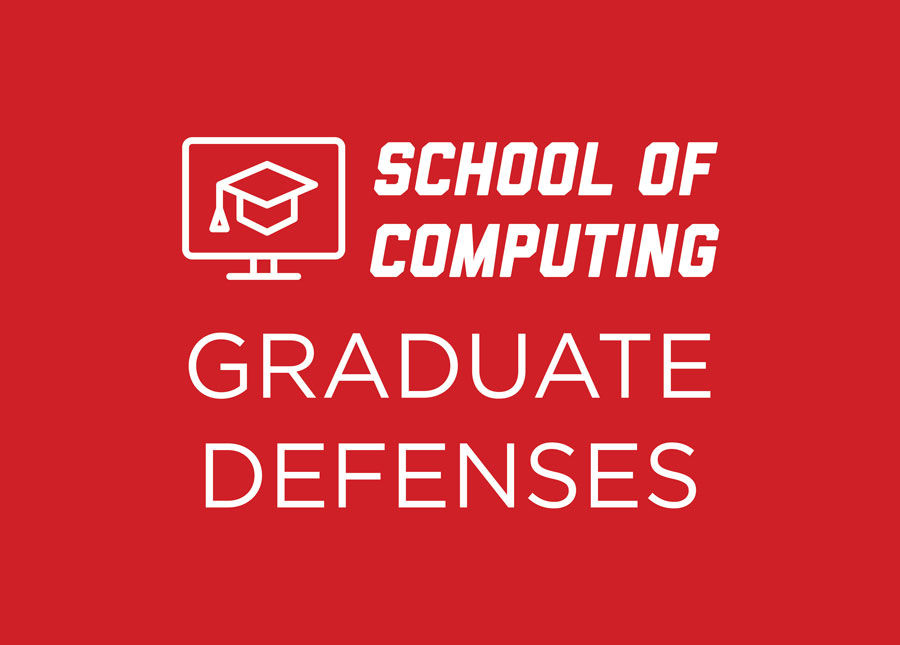
M.S. Thesis Defense: Mitchell DeHaven
Monday, November 28, 2022
11:00 AM
Zoom: https://unl.zoom.us/j/95997575153
"BEVERS: A General, Simple, and Performant Framework for Automatic Fact Verification"
Fact verification has become an important process, primarily done manually by humans, to verify the authenticity of claims and statements made online. Increasingly, social media companies have utilized human effort to debunk false claims on their platforms, opting to either tag the content as misleading or false, or removing it entirely to combat misinformation on their sites. In tandem, the field of automatic fact verification has become a subject of focus among the natural language processing (NLP) community, spawning new datasets and research. The most popular dataset is the Fact Extraction and VERification (FEVER) dataset.
In this thesis an end-to-end fact verification system is built and trained based on the FEVER dataset. Our system utilizes traditional document retrieval and pretrained transformer models to form predictions. Our system does not deviate significantly from a standard approach, however we thoroughly examine design decisions and data representations to further improve the model. We suspect other results in the literature under optimized their approaches, given that we perform much better with similar system design. Finally, our system is compared against other systems through the FEVER blind test dataset and sets a new state of the art for the task while utilizing relatively simple approaches and smaller models than other systems. Our system attains the highest FEVER score on the task, while scoring second on pure accuracy, and finally our evidence retrieval system achieves the highest recall among reported results of other systems."
Committee:
Dr. Stephen Scott, Advisor
Dr. Vinod Variyam
Dr. Ashok Samal
M.S. Thesis Defense: Jigyasa Chauhan
Monday, November 28, 2022
12:00 PM (Noon)
347 Avery Hall
"An Empirical Study on the Classification of Python Language Features Using Eye-Tracking"
Eye tracking has been an evolutionary technology in the field of software engineering. We aim to utilize this technology to understand the behavior of programmers comprehending python code. Python has been constantly evolving with time. The addition of various features and functionalities has made python flexible, more-readable and easier to adapt for multi-disciplinary software programmers. But despite of new advancements to the language, we are trying to understand whether it is being used in an efficient way. Depending on how python has been utilised in the industry, it would be helpful to understand how programmers use and classify python code. The language features help us classify how programmers are using the code. Do they understand the different paradigms of python? What is their approach to debug a certain paradigm? This paper presents the first study on how programmers analyse python code using it’s features based on an eye-tracking study. To conduct this study we collected our data samples from 30 python programmers. [Results yet to be mentioned]
Committee:
Dr. Robert Dyer, Advisor
Dr. Bonita Sharif
Dr. Rahul Purandare