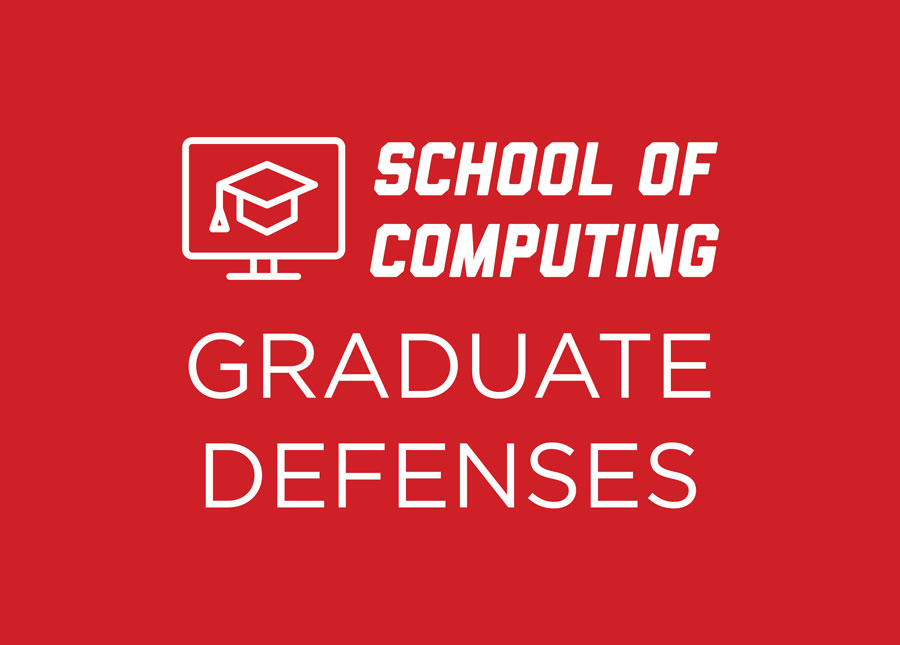
M.S. Thesis Defense: Prashant Subedi
Thursday, July 27, 2023
10:00 AM
119 Avery Hall
"Seek and Classify: End-to-end joint multi-signal detection and classification using deep learning"
The rise in the use of wireless communication has led to the problem of spectrum scarcity in licensed bands. The popularity of Internet of Things (IoT) requires innovative solutions that maximize the use of available spectrum to support the increasing number of connected devices. This thesis tackles two significant problems in wireless communication: the need for efficient spectrum sensing techniques and the scarcity of large, diverse raw in-phase (I) and quadrature (Q) datasets.
The ability to detect and classify modulation of the signals efficiently can enable cognitive radio to monitor the spectrum activity in real time and utilize unused frequencies. In this work, we propose an end-to-end framework to detect and classify narrowband signals from wideband IQ samples. The end-to-end framework reduces the sensing time for narrowband signals by 10 times. The training strategy proposed in the framework to train modulation classifiers on extracted signals increases the classification accuracy from 41% to 99%.
Given the dynamic nature of wireless channels, comprehensive datasets gathered from various environments are crucial for the success of data-driven solutions. To tackle this issue, we have designed a systematic process to collect and centralize large-sized wireless samples by using the Nebraska Experimental Testbed of Things (NEXTT) testbed. The result is an accumulation of 123 TB of raw IQ samples across a frequency range of 54 to 2,590 MHz from three distinct wireless environments.
Committee:
Dr. Mehmet Can Vuran, Chair
Dr. Stephen Scott
Dr. Shuai Nie
M.S. Thesis Defense: Fahmida Afrin
July 27, 2023
3 PM
347 Avery Hall and Zoom: https://unl.zoom.us/j/95280191765
“Spatial and Temporal Agnostic Deep-Learning-Based Radio Fingerprinting”
Radio fingerprinting is a technique that validates wireless devices based on their unique radio frequency (RF) signals. This method is highly feasible because RF signals carry distinct hardware variations introduced during manufacturing. The security and trustworthiness of current and future wireless networks heavily rely on radio fingerprinting. In addition to identifying individual devices, it can also differentiate mission-critical targets. Despite significant efforts in the literature, existing radio fingerprinting methods require improved robustness, scalability, and resilience. This study focuses on the challenges posed by spatial-temporal variations in the wireless environment. Many prior approaches overlook the complex numerical structure of the in-phase and quadrature (I/Q) data by treating real and imaginary components separately. This approach results in the loss of essential information encoded in the signal’s phase and amplitude, leading to lower accuracy. Our paper proposes several enhancements. First, we conduct extensive experiments to determine optimal pre-processing parameters, ensuring that over-optimistic conclusions about RF fingerprinting performance are avoided. Second, we treat the entire complex structure of the I/Q data as a single input to a complex-valued convolutional neural network (CVNN), thereby improving the model’s accuracy. Third, we compare transfer learning-based fine-tuning and a triplet network to address the variations introduced by the wireless environment in scenarios involving different locations and periods. We use the concept of a “device rank” metric to perform device identification with certainty based on RF fingerprinting. Furthermore, we evaluated that the Concatenated Rectified Linear Units (CReLU) activation function outperforms two other activation functions when testing cross-location and time device fingerprints.
Committee members:
Dr. Nirnimesh Ghose, Chair
Dr. Lisong Xu
Dr. Byrav Ramamurthy