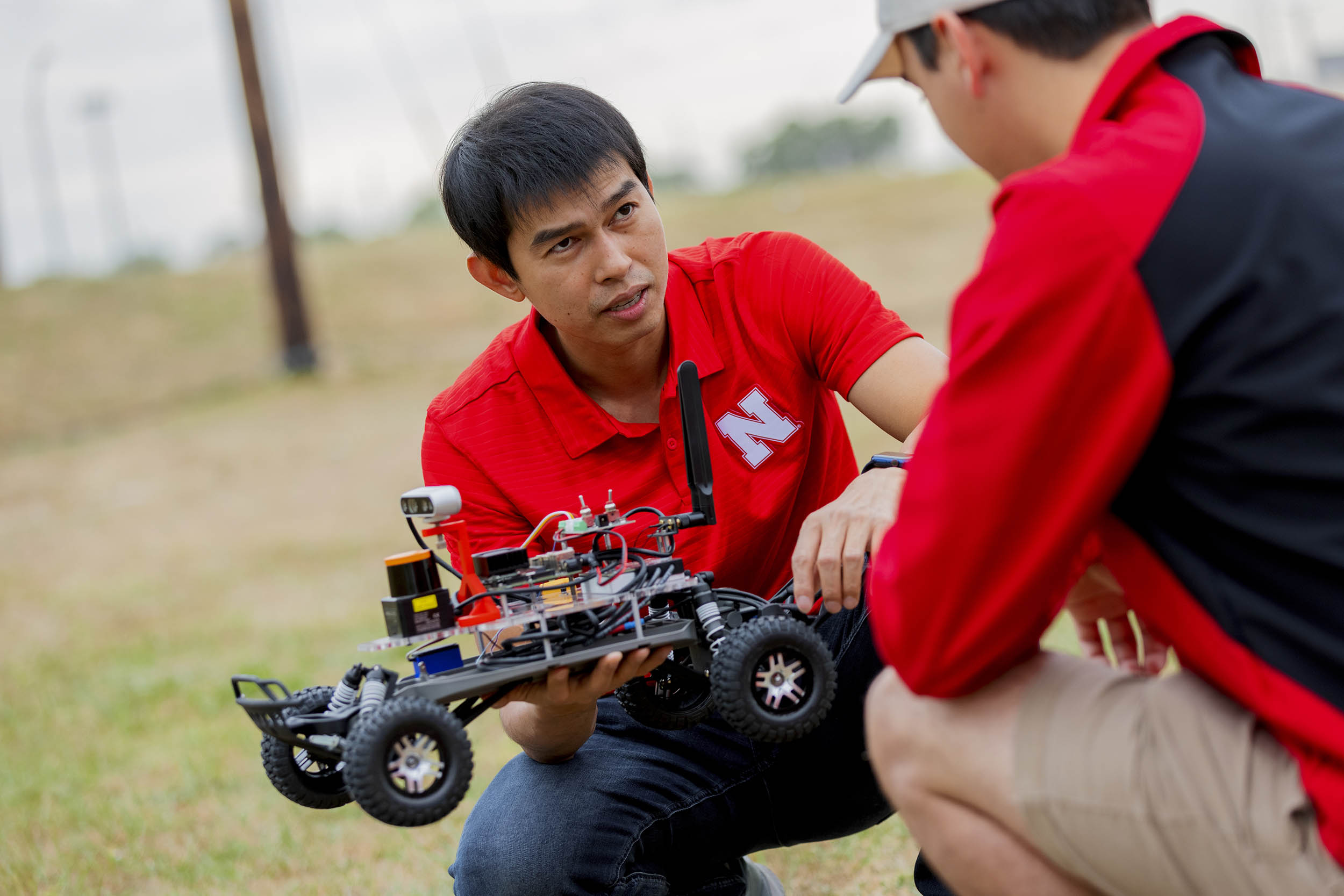
The invention of autonomous vehicles has proven to be an exciting advancement in the technology field, but many remain apprehensive about their introduction into society due to safety concerns. School of Computing assistant professor Dung Hoang Tran is working on a new solution to change that.
With the support of a new $529,041 grant from the National Science Foundation, Tran will continue his work on a verification tool that will make autonomous vehicles and other robotics machinery both safer and smarter.
Autonomous vehicles are able to operate in unfamiliar environments thanks to learning components that have been modeled after real-world scenarios and integrated into their systems. When so many components are combined with unpredictable and unprecedented factors in live environments, safety assessment and risk management can become quite challenging for engineers.
"We have to build a very complex mathematical and computational framework to be able to analyze a complex system like that,” Tran said. “When you design that type of system, the deep learning models might behave in a way that could lead your system into a scenario that you don't want.”
As a Ph.D. student at Vanderbilt University, Tran created the Neural Network Verification (NNV) tool, a type of safety verification software that is still currently and widely used by major companies in many industries, including Apple, Boeing, and Toyota. Tran's new tool will build on his previous work to provide engineers with much more comprehensive safety assessments and results.
Tran’s new tool will measure safety using both qualitative and quantitative methodologies. In addition to certifying that a system passes or fails safety tests, the new tool will also analyze the probability of risk involved to provide timely, detailed, and accurate evaluations at the system level.
“In our new framework, we can also ask, ‘If it’s unsafe, then how unsafe?’” Tran said. “It models the uncertainty of the environment, and that is going to give you the probability of a specification or a requirement being violated, which is very important for decision making or control.”
Tran’s project will use the same novel language he developed for NNV, the probabilistic star temporal logic specification language. This language allows engineers to determine the requirements necessary for the system to behave correctly and safely. Tran and his team will then work to design efficient verification techniques and algorithms that measure the system safety against the requirements.
“If we cannot prove that the system is safe, then we need to generate what we call the ‘counter example,’ or the proof that system is actually unsafe,” Tran said. “Using these counter examples, we can then return the deep learning model or change the design of the system, which can enhance the safety of the system.”
After joining the University of Nebraska–Lincoln and becoming a co-director of the Nebraska Intelligent MoBile Unmanned Systems Lab (NIMBUS) Lab in 2018, Tran began building a testbed in the lab to test robotics machinery and software. The learning-enabled F1TENTH testbed is a small-scale system used to create real-world scenarios for autonomous vehicles to evaluate their applicability, scalability, and reliability.
According to Tran, ensuring autonomous vehicle safety requires not only that they perform correctly as they’re designed, but also that they react intelligently when unplanned situations occur.
“Testing is not enough to guarantee the safety of the system when it works in the real world,” Tran said. “We want to make sure that the system itself has some internal decision-making process so that when something wrong happens inside the car, it can automatically figure out what's going to happen next, then perform some smart decisions so it can prevent other problems.”
Tran said that he hopes to continue expanding work on his tool in the future and eventually create one that could be useful for other robotics developers, like his fellow NIMBUS researchers.
“The ultimate goal is that we're going to build a software tool that everyone can use,” Tran said. “My dream is that I can build another tool that actually fits perfectly for robotics people, and they can adopt it very quickly. I believe that would make a huge impact.”