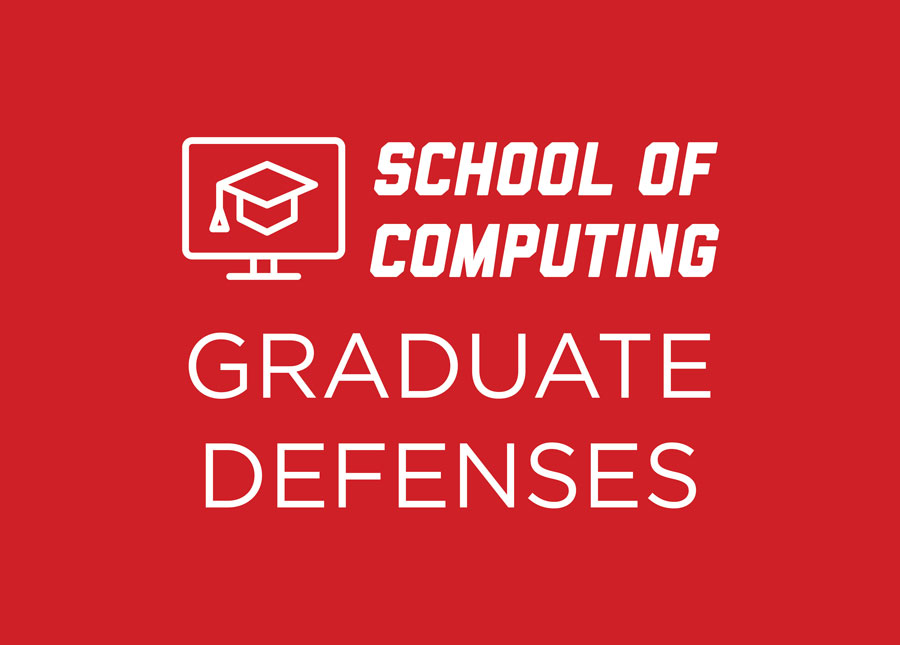
Ph.D. Dissertation Defense: Sasan Azizian
Thursday, August 1, 2024
10:00 AM CST
Zoom Link: https://unl.zoom.us/j/92613099100
“A Data-Driven Discovery System for Studying Extracellular microRNA Sorting and RNA-Protein Interactions”
Interactions between microRNAs (miRNAs) and RNA-binding proteins (RBPs) are pivotal in miRNA-mediated sorting, yet the molecular mechanisms underlying these interactions remain largely understudied. Few miRNA-binding proteins have been verified, typically requiring extensive laboratory work. This study introduces DeepMiRBP, a novel hybrid deep learning model designed to predict microRNA-binding proteins. The model integrates Bidirectional Long Short-Term Memory (Bi-LSTM) networks with attention mechanisms, transfer learning, and cosine similarity to offer a robust computational approach for inferring miRNA-protein interactions.
DeepMiRBP is implemented through two distinct architectures. The first architecture employs a Y-shaped model that uses Bi-LSTM networks and transfer learning to extract contextual relations and identify similarities between miRNA and RNA sequences. This approach leverages the power of Bi-LSTM networks to capture sequential dependencies and context within RNA sequences, while attention mechanisms enhance the model\u2019s focus on the most relevant features. Transfer learning allows the model to apply knowledge gained from a large dataset of RNA-binding proteins to the specific task of predicting miRNA-protein interactions. The second architecture improves upon the first by incorporating cosine similarity and transfer learning. This architecture includes two main components. The first component utilizes Bi-LSTM networks and transfer learning to process RNA sequences that bind to RBPs, capturing essential sequence features and embedding them into a 128-dimensional vector space. Cosine similarity is then used to assess the similarities between these sequences and miRNA sequences. The second component employs Convolutional Neural Networks (CNNs) and structural information of proteins, including Position-Specific Scoring Matrices (PSSM) and contact maps, to encode protein structures into unique vectors. Cosine similarity is applied again to evaluate the similarity between pairs of proteins, resulting in a comprehensive similarity matrix. Using DeepMiRBP, we accurately predict known miRNA interactions with recently discovered exosomal transporter proteins responsible for miRNA sorting, including AGO, YBX1, and FXR2. This capability underscores the model\u2019s potential to identify novel transporter proteins, which are crucial molecular determinants for exosome-mediated small RNA sorting and secretion, as well as other miRNA-protein interaction processes. The methodologies and insights from DeepMiRBP offer a scalable template for future research, from mechanistic discovery to cell-to-cell communication in human disease development, emphasizing its adaptability and potential for developing novel RNA-centric therapeutic interventions and personalized medicine.
Moreover, DeepMiRBP has demonstrated significant accuracy in predicting RNA-binding interactions, making it a valuable tool for studying both miRNA sorting and broader RNA-protein interactions. The model’s innovative combination of Bi-LSTM networks, CNNs, transfer learning, and cosine similarity represents a significant advancement in computational biology, providing a powerful framework for understanding the complex networks that govern cellular function.
Committee:
Dr. Juan Cui, Advisor
Dr. Hamid Bagheri, Co-Advisor
Dr. Qiuming Yao
Dr. Mohammad Rashedul Hasan
Dr. Hamid Sharif