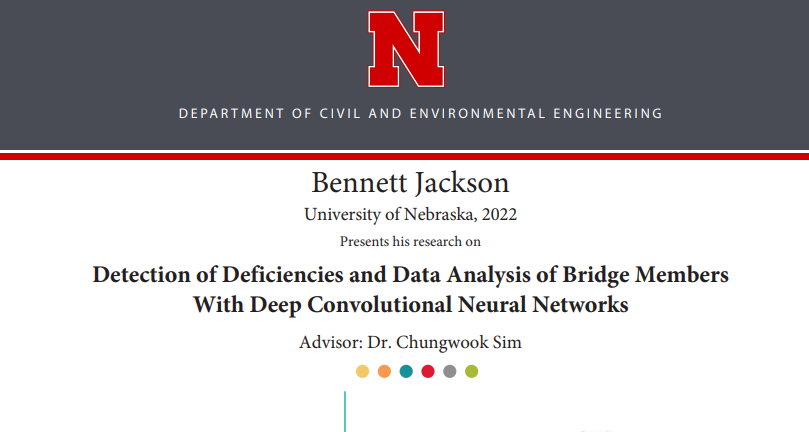
Bennett Jackson
April 25th
2:00pm-4:00pm
NH W181A
Zoom: https://unl.zoom.us/j/97574376578
Detection of Deficiencies and Data Analysis of Bridge Members
With Deep Convolutional Neural Networks
Concrete cracks and structural steel corrosion are two of the most common defects in bridges. Quantifying and classifying these defects provide bridge inspectors and engineers with valuable data for assessing deterioration levels. However, the bridge inspection process is typically a subjective, time intensive, and tedious task, as defects can be overlooked or in locations not easily accessible. Previous studies have investigated deep learning-based inspection methods, implementing popular models such as Mask R-CNN and U-Net. The architectures of these models offer certain advantages depending on the required task. This thesis aims to evaluate and compare Mask R-CNN and U-Net regarding their crack detection and segmentation performances. Both models utilize identical datasets for training and validation. These datasets are compiled from publicly available sources and from previous data collection efforts by a research team at the University of Nebraska-Lincoln. Detection accuracy is evaluated utilizing a labeled orthomosaic image of a pedestrian bridge located in Lincoln, Nebraska. Analyzing the effectiveness of the deep convolutional neural networks on a
large-scale image, rather than local images, is more akin to how traditional inspections are completed. In addition, both models are evaluated for their capabilities through surface strain analysis on the same pedestrian bridge.