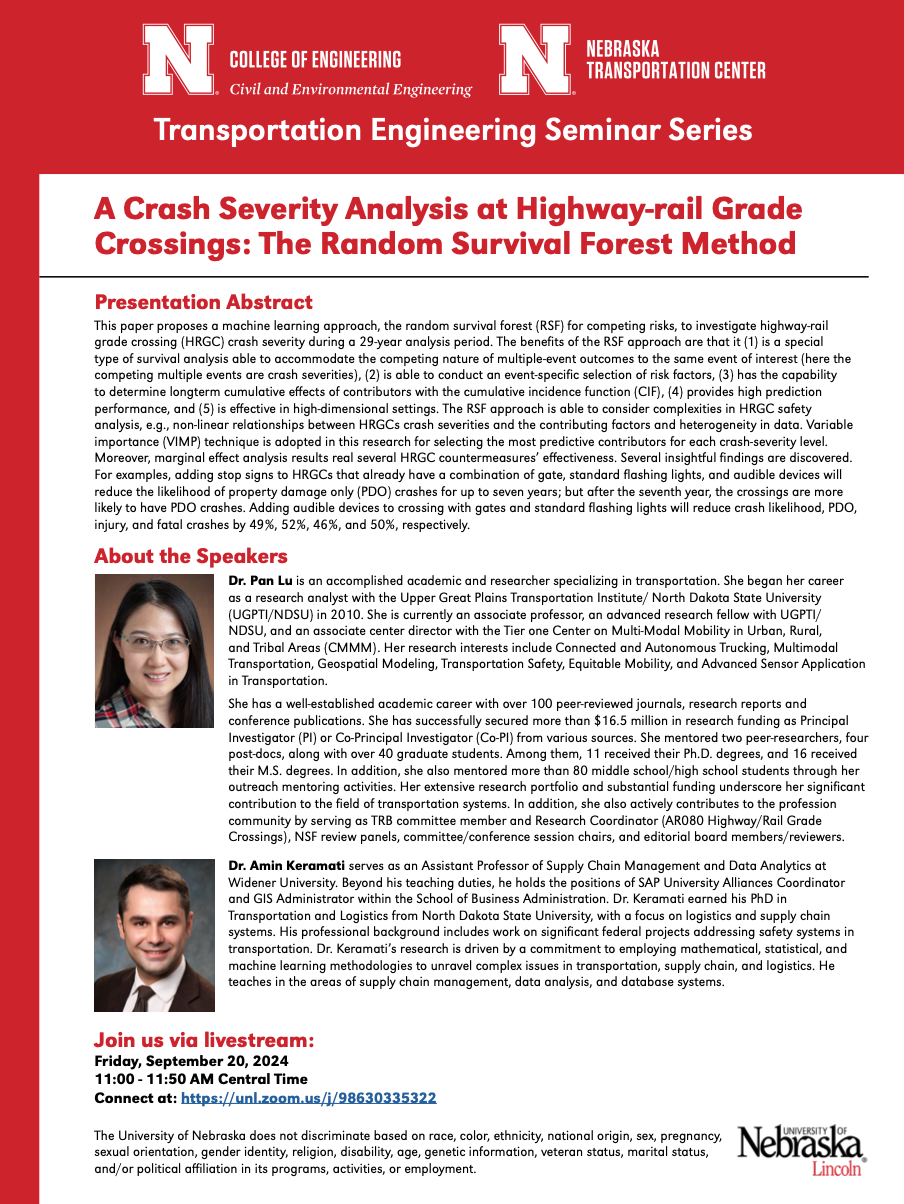
Who: Dr. Pan Lu and Dr. Amin Keramati
When: Friday, September 20, 2024, 11:00AM - 11:50AM
Where: connect at: https://unl.zoom.us/j/98630335322
Abstract:
This paper proposes a machine learning approach, the random survival forest (RSF) for competing risks, to investigate highway rail grade crossing (HRGC) crash severity during a 29-year analysis period. The benefits of the RSF approach are that it (1) is a special type of survival analysis able to accommodate the competing nature of multiple-event outcomes to the same event of interest (here the competing multiple events are crash severities), (2) is able to conduct an event-specific selection of risk factors, (3) has the capability to determine longterm cumulative effects of contributors with the cumulative incidence function (CIF), (4) provides high prediction performance, and (5) is effective in high dimensional settings. The RSF approach is able to consider complexities in HRGC safety analysis, e.g., non-linear relationships between HRGCs crash severities and the contributing factors and heterogeneity in data. Variable importance (VIMP) technique is adopted in this research for selecting the most predictive contributors for each crash-severity level. Moreover, marginal effect analysis results real several HRGC countermeasures’ effectiveness. Several insightful findings are discovered. For examples, adding stop signs to HRGCs that already have a combination of gate, standard flashing lights, and audible devices will reduce the likelihood of property damage only (PDO) crashes for up to seven years; but after the seventh year, the crossings are more likely to have PDO crashes. Adding audible devices to crossing with gates and standard flashing lights will reduce crash likelihood, PDO, injury, and fatal crashes by 49%, 52%, 46%, and 50%, respectively.