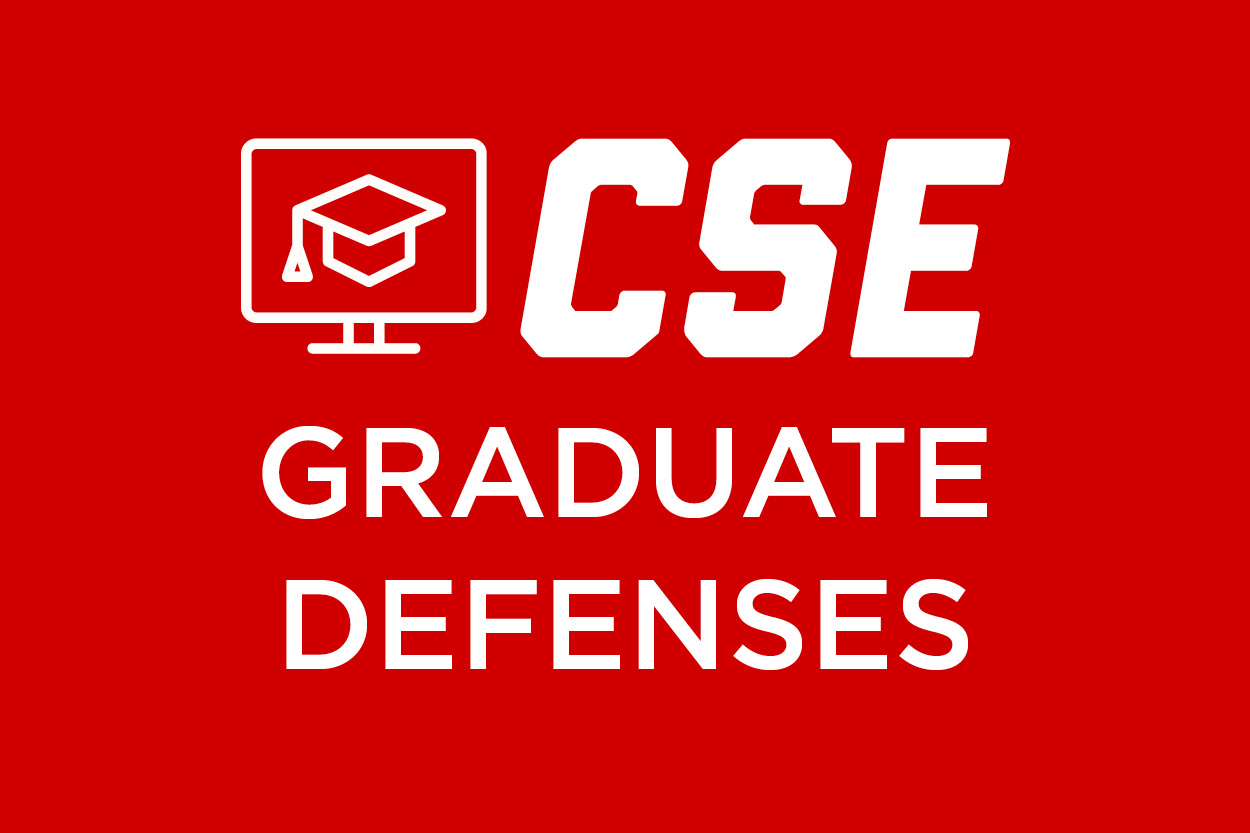
Thesis Defense: Yu Pan
Thursday, August 5, 2021
9:00 AM Central Time (US and Canada)
Zoom: https://unl.zoom.us/j/97176922143
"3D Tracking and Analysis on Multivariate Time-Varying Scientific Data"
Scientists generate data from experiments or simulations of unprecedented complexity by being equipped with advanced sensors and supercomputing techniques. The generated datasets often have vast amounts and involve hundreds or even thousands of time steps. Scientists are interested in exploring and analyzing the generated datasets and gaining insights into various phenomena. Traditionally, scientists transfer a dataset to their local machines for analysis. However, as the amount of data grows exponentially, it quickly becomes inefficient or impossible to move large-scale datasets. A common practice is in-situ data analysis, where scientists use the same supercomputer generating the raw data to conduct initial analyses and data representation generation and then only transfer important data to their local analysis machines. While in-situ data analysis can alleviate the data transmission bottleneck, it is non-trivial to generate an appropriate data representation that captures the essentials in the raw data. We address this issue from two viewpoints to modeling time-varying scientific data. The first is the Eulerian viewpoint, where we can consider a dataset as four-dimensional volumetric data in space and time. The second is the Lagrangian viewpoint, where we track a single particle through space and time and establish the dynamic equation of its locations. Based on these two viewpoints, this dissertation studies new approaches to deriving compacted data representations of large-scale time-varying scientific data in an accurate and scalable manner. First, we present a deep learning-based method that can adaptively capture the inherently complicated dynamics of temporal-spatial volumetric datasets. We train an autoencoder-based neural network with quantization and adaptation. Compared with existing methods, our method can learn data representation at a much lower compressed/uncompressed rate while preserving the details of original datasets. Second, we present particle flow, a technique that can capture the inherently complex dynamics of scientific datasets from the Lagrangian viewpoint. Our method does not rely on any feature description and comparison in traditional optical flow-based methods and can adapt to complex feature transformations across data frames. We can easily reconstruct any intermediate frames by interpolating the starting and ending frames using the resulting particle flow. Third, to better understand uncertainties introduced in data representations and derive more accurate approaches, we assess deep neural networks (DNNs) to estimate conditional probabilities and propose a framework for systematic uncertainty characterization. The gained insight can help delineate the capability of DNNs as probability estimators and aid the interpretation of the inference produced by various deep models. Finally, we generalize our Eulerian and Lagrangian viewpoints-based approach based on our uncertainty quantification framework. We trace the particles in a probabilistic manner such that we interpret each trajectory as a stochastic process. Therefore, we can describe the uncertainty for each trajectory systematically. Furthermore, we analyze these probabilistic trajectories by clustering them and comparing the patterns of various clusters. Extensive experiments and visualizations on different datasets show that our method can help scientists effectively capture and retain essentials from large-scale scientific datasets.
Committee:
- Dr. Hongfeng Yu
- Dr. Lisong Xu
- Dr. Ashok Samal
- Dr. Chi Zhang