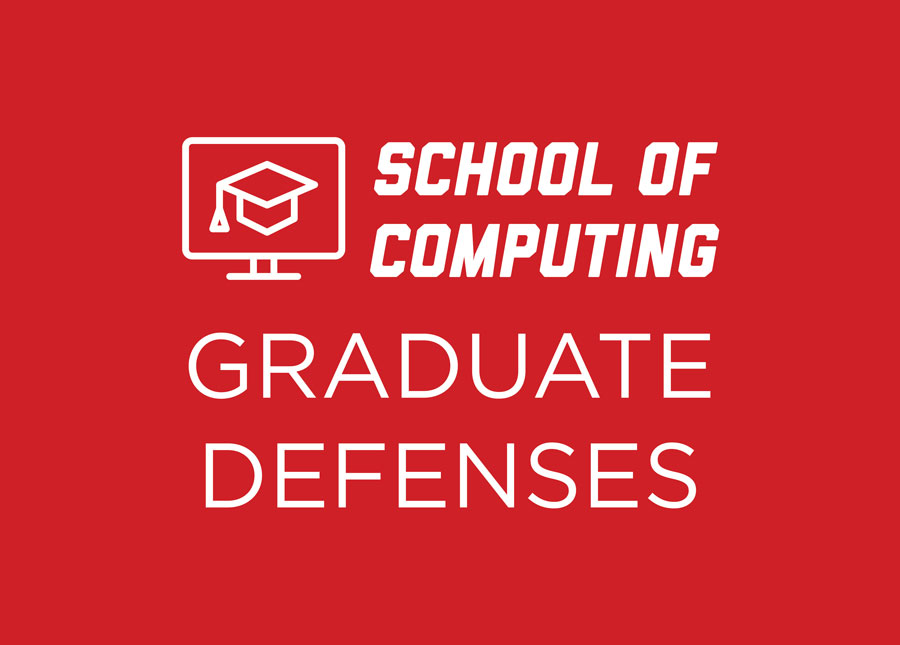
M.S. Defense: Archit Srivastava
Wednesday, July 27, 2022
1 PM
347 Avery Hall and Via Zoom
Zoom: https://unl.zoom.us/j/91905181819
"Feed Forward Neural Networks with Asymmetric Training"
Our work presents a new perspective on training feed-forward neural networks(FFNN). We introduce and formally define the notion of symmetry and asymmetry in the context of training of FFNN. We provide a mathematical definition to generalize the idea of sparsification and demonstrate how sparsification can induce asymmetric training in FFNN. In FFNN, training consists of two phases, forward pass, and backward pass. We define symmetric training in FFNN as follows-- If a neural network uses the same parameters for both forward pass and backward pass, then the training is said to be symmetric.The definition of asymmetric training in artificial neural networks follows naturally from the contrapositive of the definition of symmetric training. Training is asymmetric if the neural network uses different parameters for the forward and backward pass. We conducted experiments to induce asymmetry during the training phase of the feed-forward neural network such that the network uses all the parameters during the forward pass, but only a subset of parameters are used in the backward pass to calculate the gradient of the loss function using sparsified backpropagation. We explore three strategies to induce asymmetry in Neural networks. The first method is somewhat analogous to drop-out because the sparsified backpropagation algorithm drops specific neurons along with associated parameters while calculating the gradient. The second method is excessive sparsification. It induces asymmetry by dropping both neurons and connections, thus making the neural network behave as if it is partially connected while calculating the gradient in the backward pass. The third method is a refinement of the second method; it also induces asymmetry by dropping both neurons and connections while calculating the gradient in the backward pass. In our experiments, the FFNN with asymmetric training reduced overfitting, had better accuracy, and reduced backpropagation time compared to the FFNN with symmetric training with drop-out.
Committee:
Dr. Vinod Variyam, Advisor
Dr. Stephen Scott
Dr. Ashok Samal
M.S. Defense: Richard Maina
Wednesday, July 27, 2022
2 PM
Via Zoom: https://unl.zoom.us/j/92448367051
"Simulating Sub-Threshold Communication Channels Through Neurons"
Molecular Communication is an emerging paradigm with the potential to revolutionize the technology behind wearable and implantable devices and the broad range of functions they support, from tracking physical activity to medical diagnostics. This can be achieved through intra-body communication networks that take advantage of natural biological processes as a means of transmitting, propagating and receiving information. In this thesis we focus particularly on using the neuron as a means to facilitate information transfer for interconnected wearable or implantable devices through a technique known as sub-threshold electrical stimulation. We develop upon a prior work by introducing a linear model of the neuron that incorporates a noise model. This thesis seeks to define and evaluate a communication channel using this noisy linear model. The communication channel is tested with the basic modulation techniques amplitude shift keying, frequency shift keying and phase shift keying. Additionally, we define an operational bandwidth for this communication channel and find its maximum theoretical capacity. To verify our linear model we use the widely-used NEURON software to simulate the communication channel.
Committee:
Dr. Massimiliano Pierobon
Dr. Sasitharan Basasubramaniam
Dr. Byrav Ramamurthy