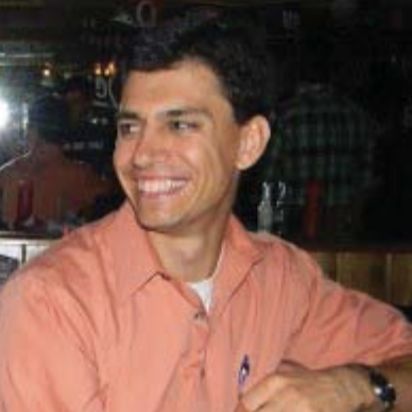
Dr. Robert A. Bridges will give a special lecture on March 13 at 4 p.m. in Avery 115.
After studying math, philosophy, and business at Creighton University, Robert (Bobby) A. Bridges pursued pure mathematics through graduate school, and obtained a Ph.D. from Purdue University with a thesis topic in the inter- section of Complex Analysis and Operator Theory. After providing applied math consultation for an engineering firm during graduate school, Bobby accepted a postdoctoral position at Oak Ridge National Laboratory (ORNL), and is currently an applied mathematician in ORNL’s Cyber & Information Security Research Group. His current research involves applying math and data science to support development of cyber security technologies.
ABSTRACT: Cyber security analysts have the unenviable task of identifying malicious activity in their network without knowledge of when or how attacks will occur. Fortunately, large-scale cyber operations have widespread collection and query capabilities for an enormous amount of logging data (network flows, system logs, alerts, ...). In general, our research focuses on developing data science (math, statistics, visualization, and computer science) tools to assist our cyber analysts. For example, using discriminant analysis to identify the progression of an identified attack, using graph theory to find suspicious network traffic patterns, and using anomaly detection to protect vehicles from signal-injection attacks are all recent applications of fairly simple mathematics targeting security applications. We’ll focus on operational difficulties of real-time anomaly detection in practice and some theorem-based solutions we’re developing. For this we assume a non-singular probability distribution has been given on a data set and propose an intuitive, principled approach to setting the alert threshold by 1. Introducing a function from the sample space to the positive reals that scores how anomalous an event is and 2. Proving that we can regulate the number of events that are flagged as anomalous, thereby circumventing the big data problem. Moreover, this approach works independently of the probability distribution, which allows comparability across distributions and needs no heuristic tuning.