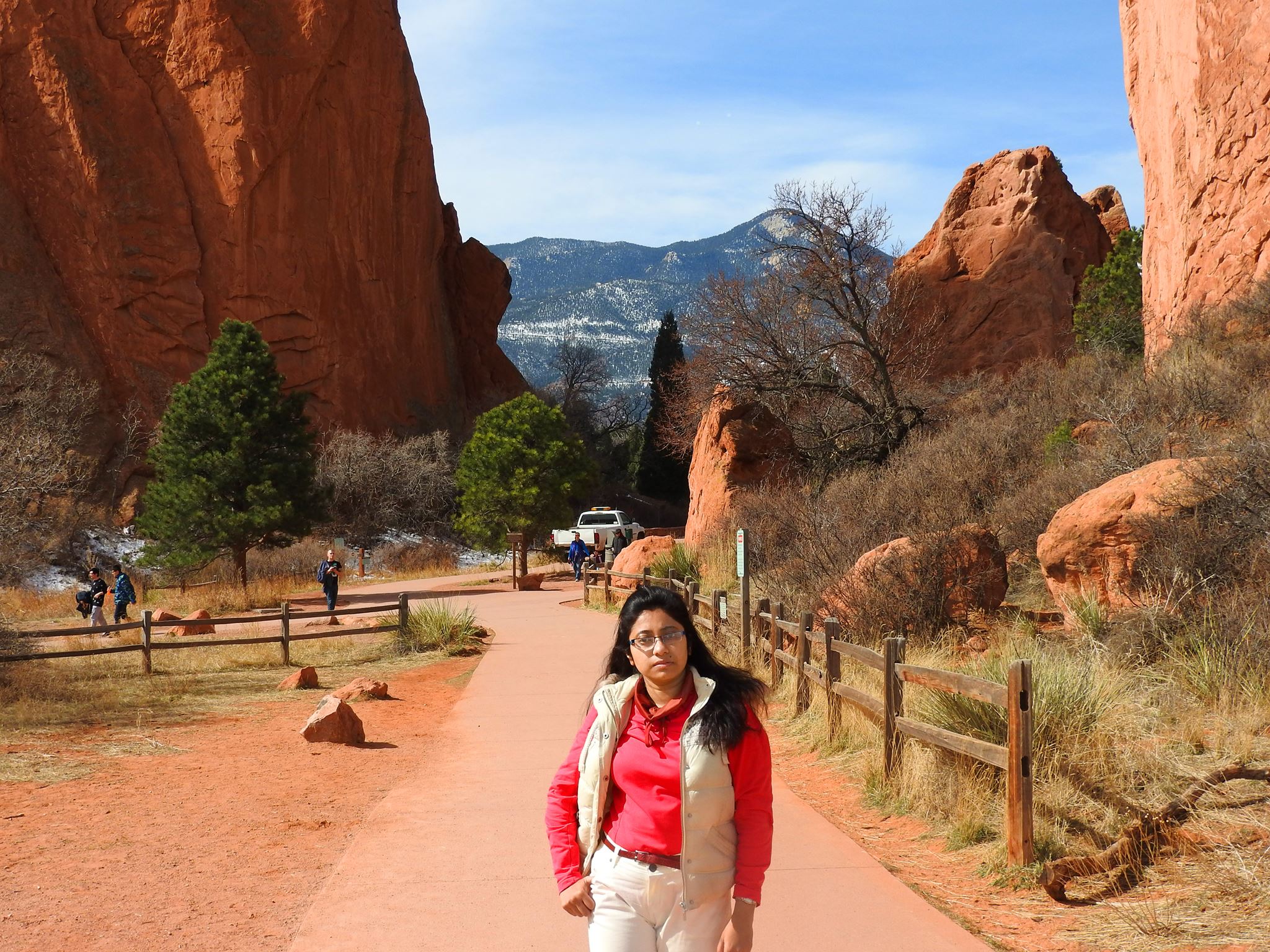
Sruti Das Choudhury, a research assistant professor in the Conservation and Survey Division at the School of Natural Resources and the Department of Computer Science and Engineering, introduced a new fall semester course, Computer Vision and Artificial Intelligence Challenges in Agriculture (NRES 498/898).
With the ease of digital image capturing facility and enormous increase in the processing power of the computers, computer vision and artificial intelligence have gained immense popularity in order to meet current and emerging issues in agriculture relating to future food security under dwindling natural resources and projected climate variability.
Imaging techniques facilitate the measurement of observable and behavioral traits of plants as a result of complex interaction between genotype and environment, i.e., phenotypes, by analyzing a large number of plants in short time interval with precision, nullifying the need for time-consuming physical human labor. Depending on the electromagnetic spectrum in which the image is captured, important information about different physiological and morphological aspects of the plant can be computed.
The course provides in-depth discussions on methodologies for image processing, including data-driven and machine learning techniques for computing plant phenotypes by considering either the whole plant as a single object or its individual components, e.g., leaves, stems, fruits and flowers. The course also covers basic shape theory with programmatic demonstrations for applications in agriculture. A method for penguin population estimation using advanced machine learning techniques using real-life image datasets captured in Antarctica is also introduced. In addition to lecture sessions, the course also covers MATLAB tutorials, hands on practices using Tableau for analyzing data, and Python coding for machine learning applications. The students’ performance have been evaluated based on graded assignments, literature review presentations and a class project.
Course features:
· understanding of recent advances in computer vision and artificial intelligence for applications in agriculture
· innovative computer vision, machine learning and deep learning methods to characterize a plant’s development, with particular focus in flower detection and counting, leaf tracking and automated detection of important events in plant’s life cycle
· discussion of a framework for plant phenotyping analysis in a multimodal, multi-view, time-lapsed, high-throughput imaging system and also presents a detailed taxonomy of plant phenotypes that can be computed by image analysis
· basic theory of data science and also practical demonstrations of Tableau as a data visualization tool
The students develop Matlab programming skill to compute phenotypes using visible light, hyperspectral and fluorescent images. Basic theory of shape analysis is also discussed and Python coding is introduced for machine learning applications.
To learn more, contact Choudhury at s.d.choudhury@unl.edu.