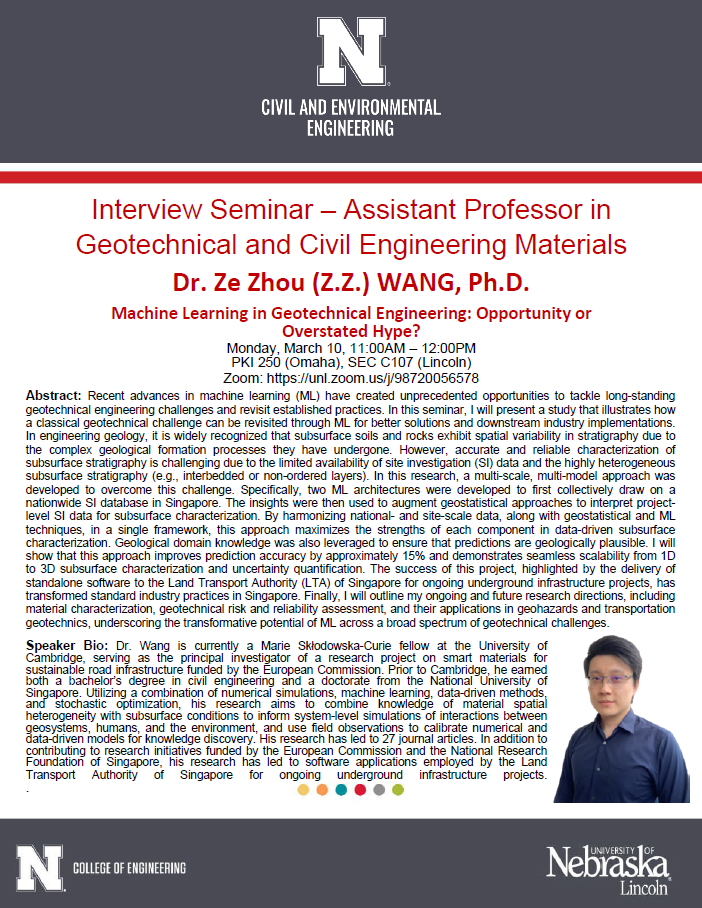
Speaker: Dr. Ze Zhou (Z.Z.) WANG, Ph.D.
When: Monday, March 10, 11:00AM – 12:00PM
Where: PKI 250 (Omaha), SEC C107 (Lincoln)
Zoom: https://unl.zoom.us/j/98720056578
Title: Machine Learning in Geotechnical Engineering: Opportunity or Overstated Hype?
Abstract: Recent advances in machine learning (ML) have created unprecedented opportunities to tackle long-standing geotechnical engineering challenges and revisit established practices. In this seminar, I will present a study that illustrates how a classical geotechnical challenge can be revisited through ML for better solutions and downstream industry implementations. In engineering geology, it is widely recognized that subsurface soils and rocks exhibit spatial variability in stratigraphy due to the complex geological formation processes they have undergone. However, accurate and reliable characterization of subsurface stratigraphy is challenging due to the limited availability of site investigation (SI) data and the highly heterogeneous subsurface stratigraphy (e.g., interbedded or non-ordered layers). In this research, a multi-scale, multi-model approach was developed to overcome this challenge. Specifically, two ML architectures were developed to first collectively draw on a nationwide SI database in Singapore. The insights were then used to augment geostatistical approaches to interpret projectlevel SI data for subsurface characterization. By harmonizing national- and site-scale data, along with geostatistical and ML techniques, in a single framework, this approach maximizes the strengths of each component in data-driven subsurface characterization. Geological domain knowledge was also leveraged to ensure that predictions are geologically plausible. I will show that this approach improves prediction accuracy by approximately 15% and demonstrates seamless scalability from 1D to 3D subsurface characterization and uncertainty quantification. The success of this project, highlighted by the delivery of standalone software to the Land Transport Authority (LTA) of Singapore for ongoing underground infrastructure projects, has transformed standard industry practices in Singapore. Finally, I will outline my ongoing and future research directions, including material characterization, geotechnical risk and reliability assessment, and their applications in geohazards and transportation geotechnics, underscoring the transformative potential of ML across a broad spectrum of geotechnical challenges.