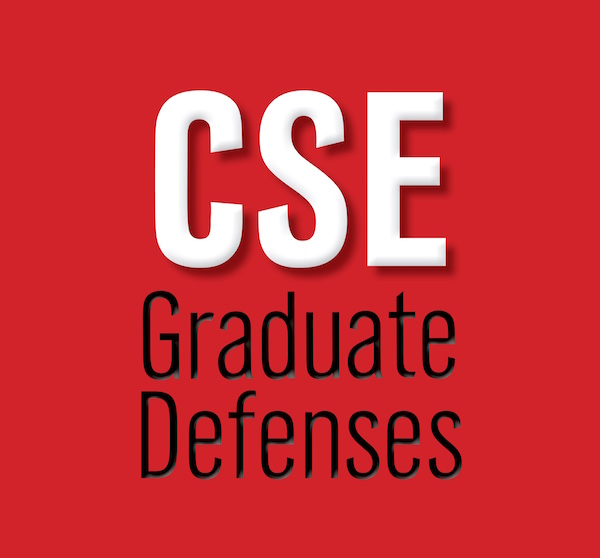
Master's Project Defense: "Distributed Energy Management in a Microgrid: A Multiagent Reinforcement Learning Approach"
Elham Foruzam
Committee Members: Dr. Leen-Kiat Soh (Advisor)
Dr. Jitender Deogun and Dr. Vinod Variyam
Friday, April 7, 2017, 2:30 p.m.
256C Avery Hall
Abstract: In this project, a multiagent-based model is used to study the distributed energy management in a microgrid (MG). The suppliers and consumers of electricity are modeled as autonomous agents, capable of making local decisions in order to maximize their own profit in a multiagent environment. For every supplier, a lack of information about customers and other suppliers creates challenges to make optimal decisions in order to maximize its return. Similarly, customers face difficulty in scheduling their energy consumption without any information about suppliers and electricity prices. Additionally, there are several uncertainties involved in the nature of MGs due to variability in renewable generation output power and continuous fluctuation of customers' consumption. In order to prevail over these challenges, a reinforcement learning algorithm was developed to allow generation resources, distributed storages, and customers to develop optimal strategies for energy management and load scheduling without prior information about each other and the MG system. Case studies are provided to show how the overall performance of all entities converges as an emergent behavior to a Nash equilibrium, benefiting all agents.