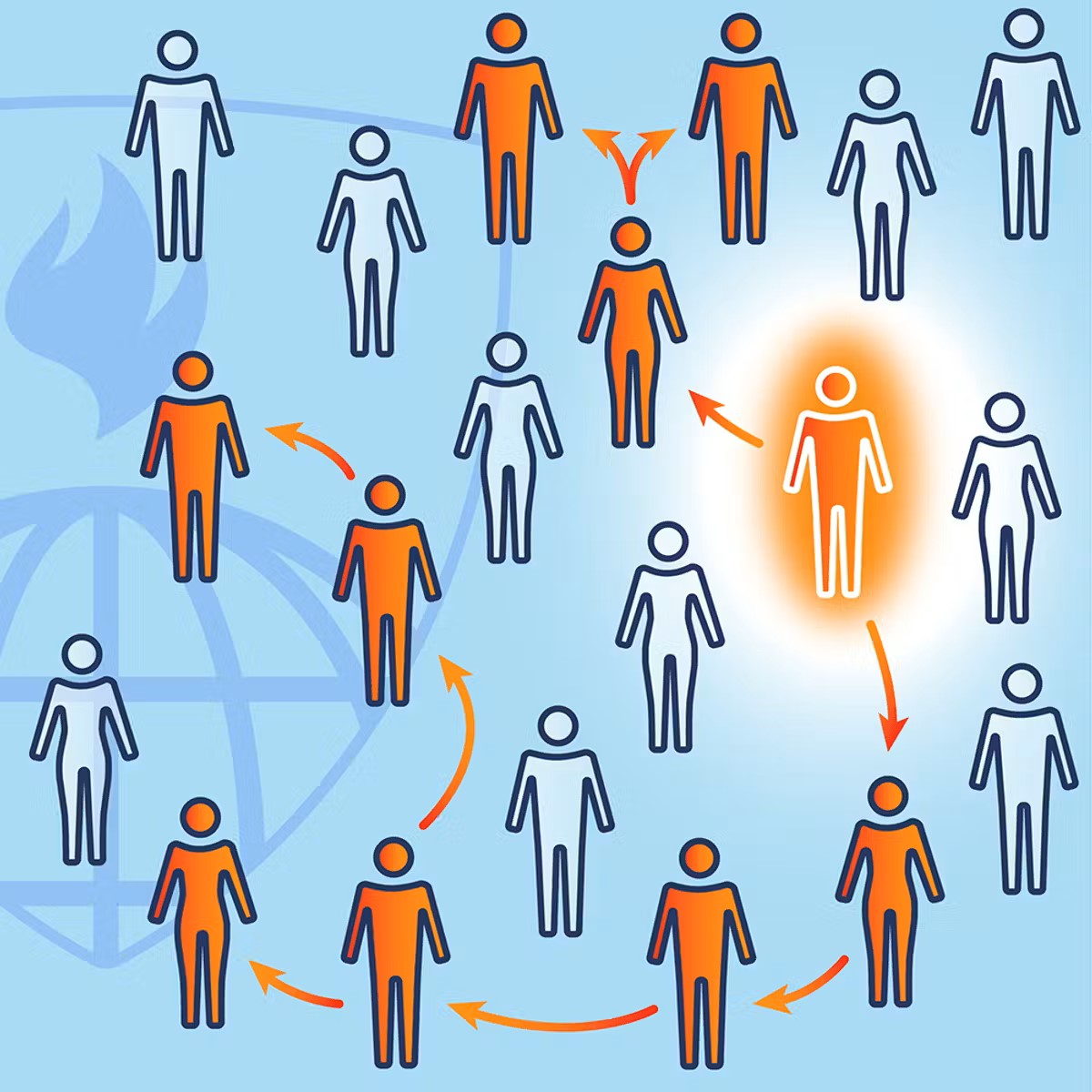
The Math In The City Miniconference this semester will be about disease models. There will be talks by six groups, three on Wednesday (May 10) and three on Friday (May 12). You can attend in person in the lecture hall Brace 206 or via Zoom at the links provided. Feel free to come to some or all the talks.
Math In The City Miniconference – Infectious Disease Modeling
Brace 206 (large lecture hall)
Wednesday, May 10, 2023, 2:30-3:45: https://unl.zoom.us/j/91429238707
Friday, May 12, 2023, 2:30-3:45: https://unl.zoom.us/j/99689367083
Wednesday, May 10th
2:30-2:55: Finding R0
Philip Chohon, Dennis Startsev, Cleve Young
R0 is perhaps the single most important number in epidemiology. It is defined as expected number of new infections that an individual will cause when there is a nearly total susceptible population. The most direct way to calculate this is through contact tracing, but other methods have been created. In this paper we explore two of those methods, one that calculates R0 from cumulative data, and another that calculates it from an ODE model.
2:55-3:20: Modeling Vampires: The Pitfalls of Mystic Falls
Abigail Hanson, Abby Heffner, Aleah Miller, Layla Montemayor
While vampire models are nothing new in the world of mathematics, the show The Vampire Diaries is yet to be explored. Using data and observations gathered from the show, we built an epidemic model to study the phenomenon of vampirism in the fictional town of Mystic Falls. We found that the outcomes in the show do not match the predictions of the model. Therefore, it became important to consider what parameters would create a functioning simulation that we felt would accurately reflect the world of The Vampire Diaries.
3:20-3:45: Fitting Covid-19 Models
Hannah Maag, Michael Bean, Spencer Schmidt
Models are a useful predictive tool. In the case of infectious disease modeling, they help policy-makers determine what measures will be needed to respond to an epidemic. Throughout the COVID-19 pandemic, various models were created in order to predict future events in regards to hospitalizations, deaths, and infections. In this work, we give a retrospective analysis of a collection of these models by fitting their parameters to data gathered from the CDC.
Friday, May 12th
2:30-2:55: The Impact of Media Attention on a Celebrity Craze
Alyssa Betterton, Jiahua Jia, Noelle Ortman, Kayla Scholz
In this talk, we study a 2012 model of the spread of “Bieber Fever” and modify the model by the addition of a skeptic class and the addition of a transmission between the skeptic class and infected class. We describe both the initial model and our modified model, comparing the outcomes.
2:55-3:20: A Disease in Two Populations with Migration
Morgan Brockner, Khai Shen Chng, David Ryckman, Jacob Spooner
In this talk, we explore how coupling two SEIR models with crossover migration might affect disease spread and how restrictions on this migration could affect peak infected. We also address how the distribution of vaccines, could affect the peak infections of the disease in question.
3:20-3:45: Twitter Misinfodemic
Brad Scheuler, Xiangyuan Su, Uyen Tran, Yuanshu Wang
The spread of misinformation is very similar to the spread of infectious diseases. During the COVID-19 pandemic in 2020, high-profile individuals posted many misleading statements on Twitter. At the same time, many media outlets refuted or promoted these statements. In this talk, we explore the impact of news media on misinformation spread via influential Twitter accounts. Our results suggest that media have a significant influence on the number of infected individuals in the long run and theoretically we can keep the impact of the misinfodemic to a minimum if we balance out the influence of pro-misinformation and anti-misinformation media.