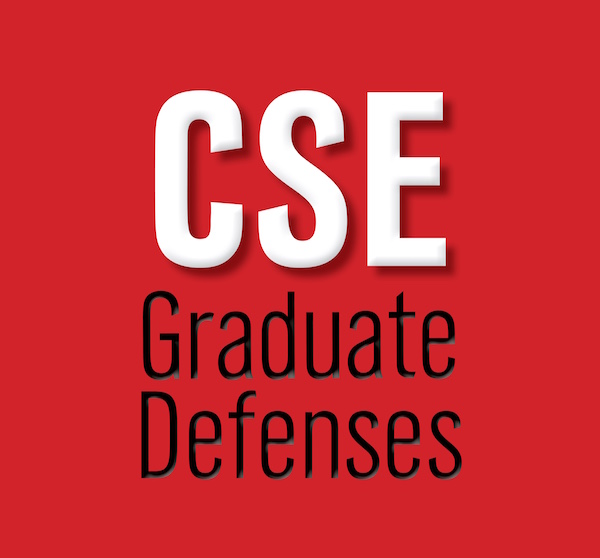
Dissertation Defense: Yu Bai
“Stochastically Dominant Access Point Selection in Wireless Local Area Network”
Committee Members: Dr. Mehmet Can Vuran and Dr. Steve Goddard (Co-Advisors)
Dr. Lisong Xu and Dr. Demet Batur
Friday, November 30, 2018, 10:00 a.m.
103 Avery Hall
Abstract: The rapid increase in the number of WiFi-enabled devices leads to the challenge of access point (AP) selection in enterprise wireless local area networks (eWLANs). Default vendor AP selection implementation results in over- and under-utilization of APs when devices cluster, and providing stochastic QoS guarantees for different applications becomes a major challenge. To this end, in this dissertation, AP selection algorithms are devised. More specifically, a stochastic network calculus concept called “?-effective service curve” is extended into “?-effective service curve”. It is proven that comparing the ?-effective service curves of two APs is sufficient to select the AP providing better QoS. The proof does not require steady-state quantile observations as in previous available bandwidth estimation techniques, therefore, transient behaviors can be compared. Utilizing this result, two AP selection algorithms, named STochastically dominant Access point selection algoRithm (STAR) and Mean-Dominance-based STAR (MD-STAR), are developed. STAR uses first-order stochastic dominance (FSD) tools from decision theory to compare quantile performance of delay in an effort to provide stochastic QoS guarantees. MD-STAR utilizes the concept of mean dominance (MD) to select the AP, which provides good average case performance with less overhead. Extensive simulations show both algorithms outperform state-of-the-art AP selection solutions both on the delay performance and stability. To further improve user’s QoS, STAR/MD-STAR selector, which relies on the calculation of remaining airtime difference, is proposed to determine which protocol to activate.
Master’s Project Defense: Kan Liu
“A New Analytical Pipeline for MicroRNA Splicing Discovery Based on Sequencing”
Committee Members: Dr. Juan Cui (Advisor)
Dr. Jitender Deogun and Dr. Chi Zhang
Monday, December 3, 2018, 2:00 p.m.
112 Schorr Center
Abstract: MicroRNAs (miRNAs) are a class of short single strand RNA molecules predominantly found in eukaryotes. Being involved in many major biological processes, miRNAs can regulate gene expression by enhancing mRNA degradation or inhibiting the translation. Current miRNA annotation has been largely hindered by the imprecision of miRNA splicing which introduces severe variability in sequencing-detected miRNA products and their corresponding downstream gene regulation. Although high-throughput small RNA-seq can produce unprecedented quantitative readouts for miRNA expression analysis, existing standalone tools usually do not consider comprehensively on miRNA splicing variations. To advance our understanding of miRNA splicing and identify differential splicing variations across different biological conditions with strong statistical power, we proposed a new bioinformatics pipeline, named GMAim, to enable novel miRNA analyses including read cataloging, expression quantification, imprecise splicing detection and categorization. Results of comparative analysis on an Arabidopsis thaliana case study showed that our pipeline outperforms existing state-of-the-art methods in term of improved accuracy in read counting and categorization and reduced computation time.